How Artificial Intelligence Impacts Product Life Cycle Management
- Coral Cubs
- Apr 10, 2024
- 3 min read
Executive Summary
Product Life Cycle Management (PLM) plays a vital role in ensuring a product's success, from conception to disposal. However, the ever-increasing complexity of products and the growing volume of data associated with them pose significant challenges for traditional PLM approaches. Artificial Intelligence (AI) offers a transformative solution, streamlining processes, enhancing decision-making, and ultimately driving product innovation and profitability.
This whitepaper explores the multifaceted impact of AI on various stages of the PLM process, highlighting its potential benefits and considerations for implementation.
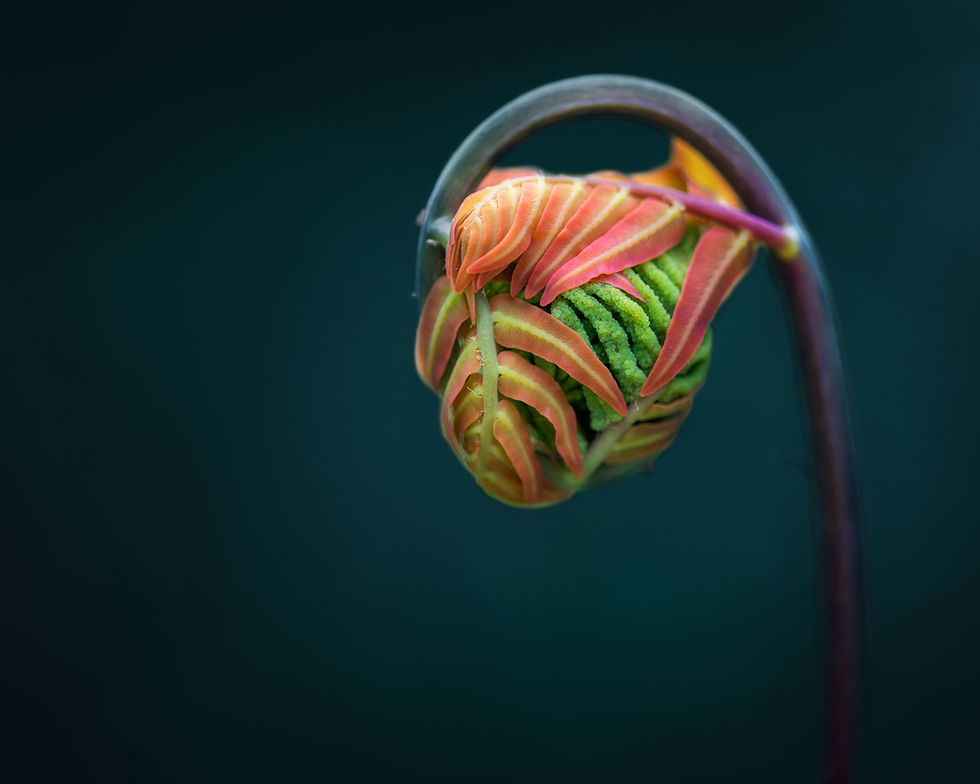
The Evolving Landscape of PLM
Modern PLM encompasses much more than just managing product data. It involves a complex interplay between engineering, design, manufacturing, marketing, and customer service functions. Here are some key challenges faced by traditional PLM systems:
Data Overload: The sheer volume of data generated throughout the product lifecycle can be overwhelming, hindering effective analysis and decision-making.
Disparate Systems: Information often resides in siloed systems across different departments, creating communication gaps and inefficiencies.
Limited Insights: Traditional PLM systems may struggle to extract actionable insights from vast amounts of data, hindering proactive problem-solving and optimization.
AI: Revolutionizing PLM
AI offers a powerful toolkit for addressing these challenges and propelling PLM to a new level of efficiency and effectiveness. Here's how:
1. Design and Development
Generative Design: AI can generate new design options based on specific criteria, accelerating the design process and fostering innovation.
Predictive Analytics: Machine learning algorithms can analyze historical data to predict potential design flaws or production issues, enabling proactive solutions.
Material Optimization: AI can analyze material properties and suggest optimal materials for product performance and cost efficiency.
2. Manufacturing and Production
Predictive Maintenance: AI algorithms can analyze sensor data from machines to predict potential failures, allowing for preventative maintenance and minimizing downtime.
Production Optimization: AI can optimize production schedules, resource allocation, and quality control processes based on real-time data analysis.
Robotics and Automation: AI-powered robots can automate repetitive tasks, improving production efficiency and consistency.
3. Supply Chain Management
Demand Forecasting: AI can analyze historical sales data and market trends to predict future demand more accurately, ensuring optimal inventory levels and avoiding stockouts.
Risk Management: AI can identify potential disruptions in the supply chain and suggest mitigation strategies, improving supply chain resilience.
Logistics Optimization: AI can optimize transportation routes, delivery schedules, and warehouse operations for improved efficiency and cost savings.
4. Marketing and Sales
Customer Segmentation: AI can analyze customer data to segment audiences and personalize marketing campaigns, leading to higher engagement and conversion rates.
Product Recommendations: AI can recommend products to customers based on their past purchases and browsing behavior, driving sales and customer satisfaction.
Price Optimization: AI can analyze market data and customer behavior to suggest optimal pricing strategies for maximizing profitability.
5. After-Sales Service
Predictive Maintenance: AI can analyze product usage data to predict potential failures and enable proactive customer service, minimizing downtime and enhancing customer experience.
Chatbots: AI-powered chatbots can provide 24/7 customer support, answer basic queries, and escalate complex issues to human agents.
Sentiment Analysis: AI can analyze customer feedback to identify areas for product improvement and ensure customer satisfaction.
Considerations for Implementing AI in PLM
While AI offers significant benefits for PLM, successful implementation requires careful consideration of several factors:
Data Quality: Robust AI models require high-quality, clean data. Organizations must prioritize data governance and ensure data accuracy.
Talent and Expertise: Integrating AI requires a skilled workforce that understands both PLM and AI principles. Training and upskilling programs are crucial.
Ethical Considerations: AI algorithms can perpetuate biases if not properly designed. Organizations must implement ethical frameworks to ensure non-discriminatory decision-making.
Wrap Up
AI is poised to revolutionize PLM, transforming it from a reactive to a proactive and data-driven discipline. By harnessing the power of AI, companies can streamline processes, gain deeper insights, and optimize product development, manufacturing, and customer service throughout the entire product lifecycle. The future of PLM is intelligent, and those who embrace AI will be well-positioned to achieve a significant competitive advantage.
Comments